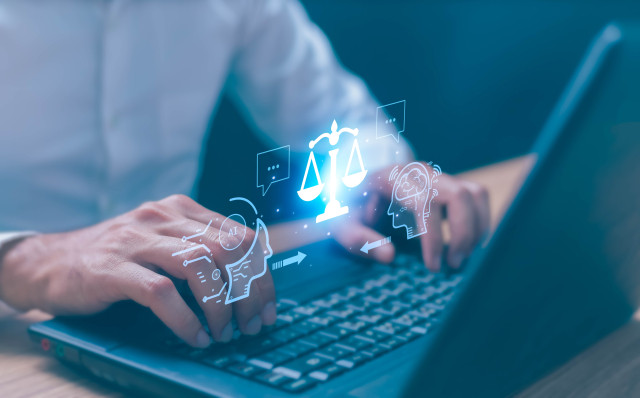
A Matter of Fairness
Why is the issue of fairness and bias relevant in both the P/C and broader insurance industries?
We’re in an era with heavier reliance on data, and more complex models to investigate that data and to gain insight from it. As the amount of data and the complexity of models continue to grow, we find that there’s greater societal concern for transparency as to what insurance companies are actually doing with respect to data and models. There’s fear that the use of this information and related modeling techniques are increasing the risk of potential hidden bias in how we use and implement these model insights. That is coupled with a current environment in which discussions are taking place with respect to fairness associated with race.
How have regulations changed in recent times? What are recent regulatory trends?
Regulators are actively asking insurance companies to evaluate the potential for bias or disparate impact in their data and modeling practices, and to contribute to mitigation if unfair bias is found. Last December, the National Association of Insurance Commissioners issued a bulletin on artificial intelligence, which has been adopted by 15 jurisdictions through the end of the first half of this year. In addition, there are unique efforts taking place in Colorado since 2021, and New York over the last few years— attempting to address the issue of evaluating potential unintended bias within the insurance models, and if found, how to mitigate the bias.
What are the challenges in defining fairness?
One of the biggest issues is that a lot of quantitative metrics can be associated with whether or not a model is fair. In the insurance industry, the existing tension is predominantly between the idea of a model, for example, predicting premium, and whether or not that premium, on average, varies across race or ethnicity. In addition, because we know that the premium is meant to reflect potential for losses, there’s also a desire for the model to accurately predict a premium that is based on losses. Because these two measures can be in tension, it is possible for a model to accurately reflect losses yet be different across different races.
How do you balance fairness regulation and the need for competitive pricing?
The problem with trying to develop equity in pricing with a sense of accuracy creates difficulty. We create situations in which, in a competitive environment, companies endeavor to price accurately. They may get a certain amount of comfort knowing that the mix of business in the portfolio may change, but because the model is relatively accurate for the policyholders, it will continue to be accurate as the mix changes. Regulation may be considered disruptive in terms of constraining how accurate that model can be. Regulation may introduce a level of subsidization into companies’ pricing schemes. Because different companies have different portfolio mixes, the way the subsidization happens will vary. There’s need and concern for balance that must be kept in mind as insurers look for equitable pricing and ways to help and support underserved communities. That needs to be coupled with an insurance environment in which more companies are able to write and offer products offering competitive pricing for those communities.
(published in Best's Review by AM Best)