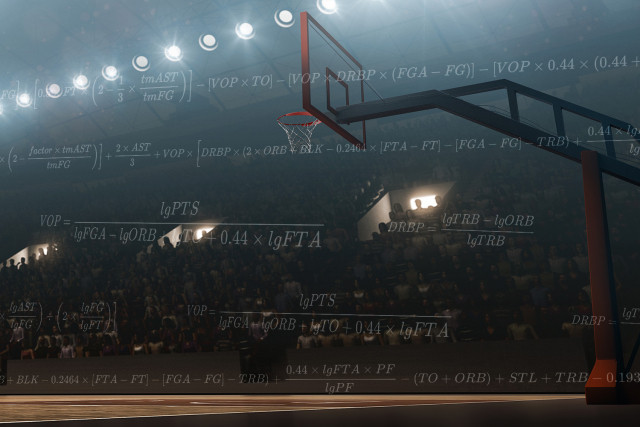
Cutting Down the Net
In 2018, the NCAA introduced a new tool to help evaluate and select teams for its annual NCAA tournament, March Madness. The NCAA Evaluation Tool (NET) and metric was designed as a way to rank NCAA basketball teams using various criteria such as strength of schedule and quality of wins or losses (akin to rating variables). It replaced the Ratings Percentage Index (RPI) that the NCAA had used for almost 40 years.
After their introduction, the NCAA’s NET rankings were heavily scrutinized by sports reporters and the general public and were not universally well-received.
Nate Silver of the website FiveThirtyEight said, “[The NET rankings are] the worst rankings I’ve ever seen in any sport, ever…the NCAA came up with something that doesn’t reflect methodological best practices and which doesn’t make sense.”
A handful of teams that didn’t belong found themselves ranked in the Top 25 upper echelon of teams, an inaccuracy clear at a glance to any avid college basketball fan. Many notable individuals in the college basketball realm expressed concerns, while others were more forgiving early on. These concerns seemed to be overreactions to a model that places too much credibility on immature data, since the NCAA did not start the season with a preseason benchmark, or an “a priori expectation.”
NCAA Senior Vice President of Basketball Dan Gavitt said of the development of the NET metric, “There was no goal or intent, in any way with any segment of the game, to benefit or not, populations of teams.”
(Gavitt’s comment may seem grossly familiar to anyone with knowledge of the fourth Property and Casualty Ratemaking Principle that states that a rate is reasonable if it is not unfairly discriminatory.)
The NCAA’s NET ranking formula is a mixture of results-based statistics and predictive algorithms. In the context of the insurance industry, this is synonymous to incorporating experience rating (results-based) and using predictive variables to produce rates for insureds.
How might the development and implementation of the NCAA’s new metric relate to the introduction and use of new models in the insurance industry?
One variable in the NET model is quality of wins, similar to strength of schedule. Each win is segmented into four quadrants based on the perceived quality of the opponent. This variable is of specific interest since games are continuously re-evaluated as the season progresses. In fact, this variable is comparable to development on known claims – a component of incurred but not reported (IBNR) claims in insurance.
- Total IBNR = Pure IBNR + IBNER
- Pure IBNR = Things that haven’t occurred yet (all of the games that have yet to be played)
- IBNER = Development on known claims (we know the results of games to date, but the quality of the opponent is subject to change once more information is gathered)
The presence of IBNR yields uncertainty. For example, suppose your favorite team beats a conference rival on day one of the season. At the time of the event, this seems like a great win. However, the team you beat proceeds to lose every game for the rest of the season. Eventually that win at the beginning of the season doesn’t seem all that impressive.
NET rankings are the most important and prominent metric for evaluating college basketball teams. But, it is important to note it is not the be-all and end-all of rankings. There are many other metrics available to value and rank teams (KenPom, Sagarin Rankings, KPI, BPI, etc.). A savvy consumer of any model—used for college basketball or in insurance—should understand which methods introduce biases and for what reasons, such that a final and informed decision can be made when deciding how to use the results from each model.
The NCAA could have taken notes from actuaries in how to properly introduce a new model. That is, there must be some balance between 1) making model results available to the public to encourage transparency and prevent a black-box mentality; and, 2) publishing the model results with proper communication and documentation. Our industry generally excels at this type of education and communication.
The NCAA case study also illustrates the futility of actuarial conspiracy theorists asking if actuaries will become obsolete. It isn’t unrealistic to argue that computer models improve efficiency and eliminate human bias. The best possible world, however, is a combination of humans and computers. Humans possess significant information to support results from a computer. A priori expectations are far from perfect, and over time, I would suggest that the reliability of a model greatly outperforms that of their human counterparts. But, humans will be able to infer, interpret and add context to model outputs – something the NCAA mostly neglected to do.